Reporting & Forecasting Development in Casual Gaming
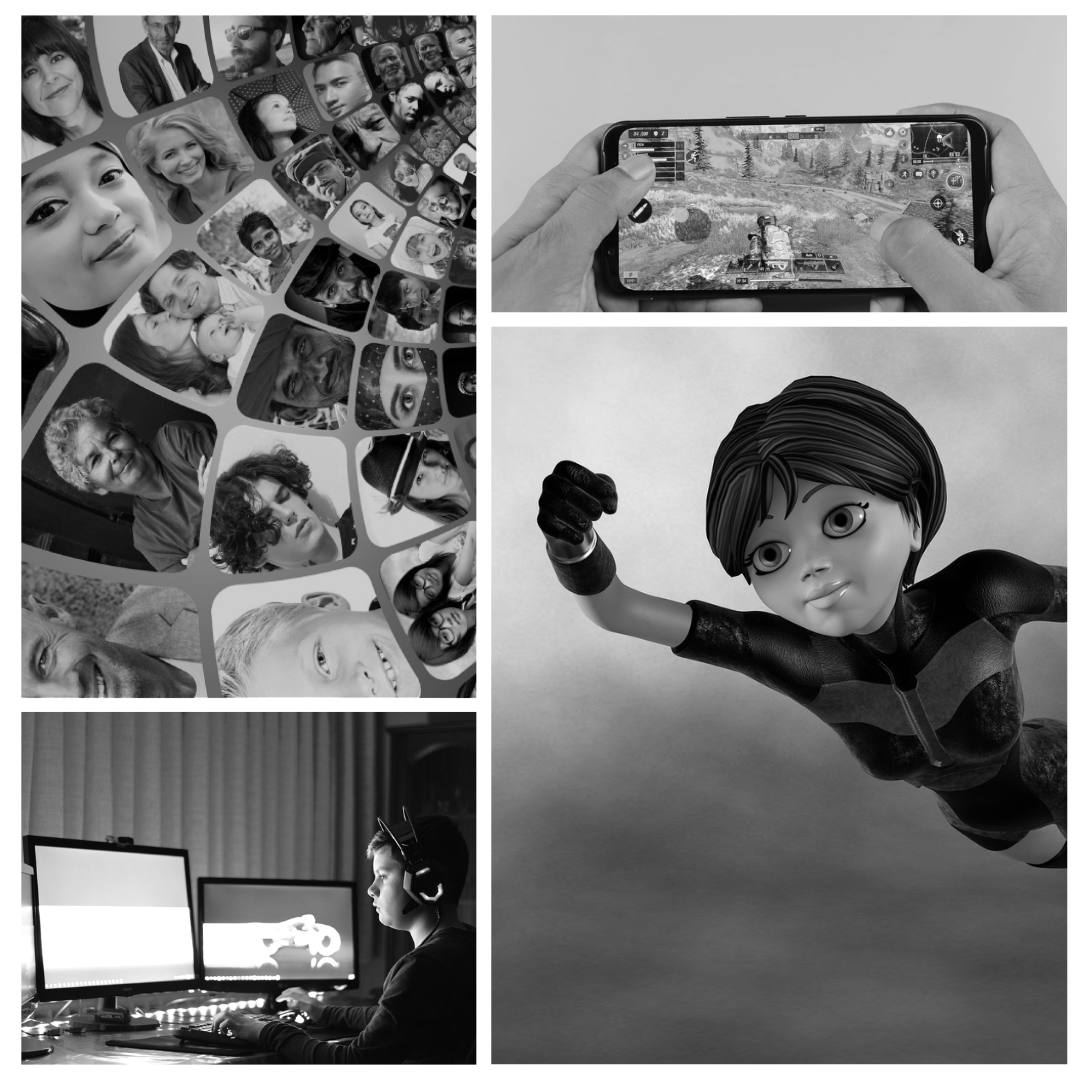
Objective
For the gaming company European Games Group, we developed and implemented three distinct user forecasting systems. These models included:
-
Revenue forecasting for past and future user cohorts
-
User activity prediction
-
Purchase probability forecasting at the individual user level
The main goal of the project was to help identify marketing campaigns and channels that attract high-value players at the lowest cost.
Additionally, revenue per game and country needed to be predicted in order to support cash flow planning.
We applied a predictive model originally adapted from use in the mobile gaming sector.
About Our Case Study Partner
The European Games Group offers an alternative to traditional publishing or the risks of self-publishing in the gaming industry. They pave the way for the next generation of independent-minded developers, providing a strong partnership to scale games in the ever-evolving and highly competitive Free2Play market.
Model 1 – Ratio-Based Forecasting Model
The ratio-based model analyzes historical data to identify trends in relative revenue development and applies these trends to new user cohorts.
By calculating aggregated weekly revenue sums and their percentage change over time, the model determines the average weekly growth rate and applies these growth factors to forecast future revenue for newer cohorts. Since the goal is to forecast one year ahead, the model requires at least one full year of training data to perform reliably.
One advantage of this model is its cross-game applicability: growth factors calculated from Game A can be applied to Game B to make revenue forecasts, assuming similar monetization behavior.
Model 2 – Purchase Probability Model
The second model focuses on estimating the individual user's likelihood to make a future purchase. Based on historical data from similar user profiles (e.g., same region, platform, behavior), the model calculates a "next purchase probability", represented as a value between 0 and 1.
While this model is not ideal for predicting aggregate revenue at the game level, it is useful for user-level predictions and targeted retention or monetization strategies. It also requires a large amount of user data to generate stable and reliable predictions.
A major benefit of this model is that it can be used across different games, as it only depends on the historical user profiles from a given game.
Model 3 – User Retention Forecasting Model
The user retention model analyzes historical data to fit various functions (e.g., linear, power, exponential) to both the number of active users and the revenue per active user.
By multiplying these two factors, the model generates a 365-day revenue forecast for each cohort.
Since the goal is to project one year ahead, this model requires a high level of data granularity and density.
Large fluctuations in user activity — such as re-engagement spikes or special in-game events — can significantly reduce the model's accuracy.
The retention model can be used across different games by applying fitted functions from Game A as training data to Game B.
This allows for revenue forecasting even if a full year of data is not yet available for Game B.
However, the decision regarding which game’s data should be used for training must be made by the user of the model.
It’s important to note that applying training data from one game to another typically results in less accurate predictions compared to using the game’s own historical data.
Looking to develop a custom forecasting model?
Why Us?
Broad Tech Stack
Depending on your database system, as well as your backend and frontend setup, we can develop solutions that are tailored to your unique requirements. We have the expertise to build custom solutions using a wide variety of programming languages and technologies.
API Expertise
We have in-depth experience working with a wide range of APIs — especially those from Google and Meta — and have already implemented numerous custom solutions.
In principle, we can integrate and utilize almost any API, adapting it precisely to your system and goals.
Forecasting Models
We have developed several proprietary forecasting models using machine learning in the past. In addition, we can enhance or extend existing systems to improve forecasting accuracy and adaptability.